A Better Way to Predict Consumer Choices
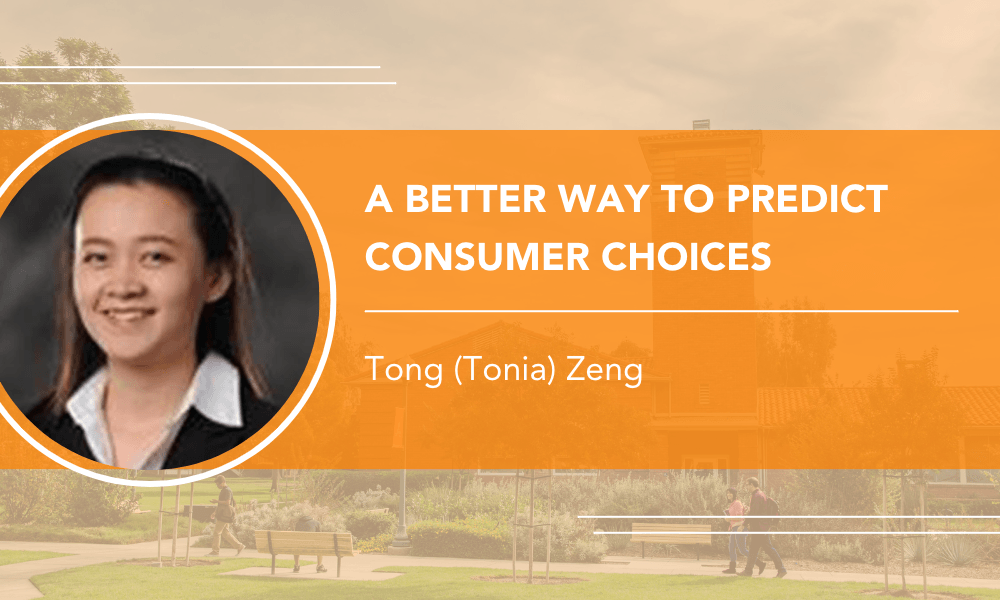
The generalized multinomial logit (GMNL) model is a tool used to study people’s preferences and predict their decisions in a variety of fields. For example, a marketing research firm may use a GMNL model to predict which features are most important to consumers when purchasing a car: fuel efficiency, reliability, performance, or some combination? The firm could collect data on the choices made by a sample of car buyers, and use the GMNL model to identify the features that have the greatest impact on consumer choice. This information could then be used by the car manufacturer to inform their product design and marketing efforts in the future.
However, there has been little research on the efficiency and accuracy of this model estimator. University of La Verne Professor Tong Zeng proposes using a more efficient methodology called the frequentist model averaging (FMA) estimator to improve the efficiency and accuracy of GMNL estimates.
The basic idea behind FMA is to combine the estimates from multiple GMNL models in order to produce a more accurate and precise estimate of the underlying consumer behavior. This is done by weighting the estimates from each individual GMNL model according to their relative performance, so that the overall estimate is more representative of the underlying data. By using FMA, researchers can more accurately predict consumer behavior. Dr. Zeng’s research did in fact confirm that using the FMA estimator improved the percentage of correct predicted choices by 10% compared to the results with GMNL estimates.
This research has been published in the journal Computational Statistics.DOI:10.1007/s00180-022-01306-4.